How Does Artificial Intelligence Work
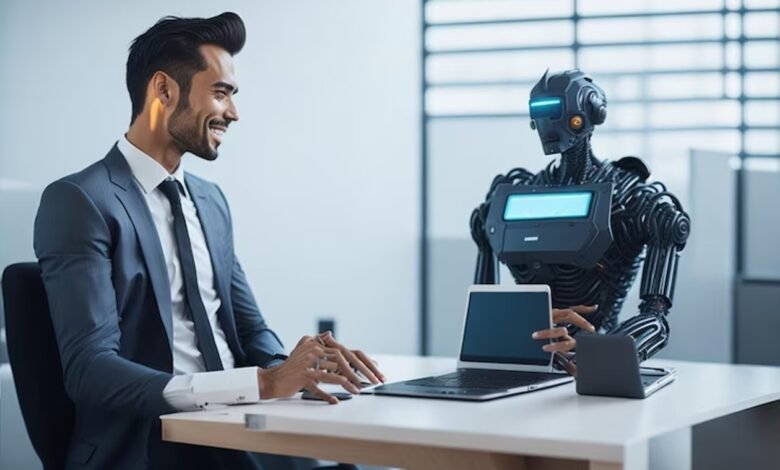
Artificial Intelligence (AI) is a revolutionary field that has been making waves in various industries, from healthcare to finance and beyond. But have you ever wondered how AI actually works? In this comprehensive guide, we will delve deep into the inner workings of AI, demystifying the complex algorithms and processes that power this incredible technology. By the end of this article, you’ll have a clear understanding of how AI processes data, learns, and makes decisions. So, let’s embark on this journey to unravel the secrets of Artificial Intelligence.
Artificial Intelligence Work
Artificial Intelligence, often abbreviated as AI, is the simulation of human intelligence in machines that are programmed to think and learn like humans. It encompasses a wide range of technologies and processes that allow machines to perform tasks that typically require human intelligence, such as understanding natural language, recognizing patterns, and making decisions.
The Basics of Machine Learning
What is Machine Learning?
Machine Learning (ML) is a fundamental component of Artificial Intelligence (AI) that enables computers to learn from data and make predictions or decisions without being explicitly programmed for a specific task. It’s like teaching a computer to recognize patterns and make sense of information, much like how humans learn from experience.
Read More: The Impact of Artificial Intelligence on SEO 2023
Types of Machine Learning
There are two primary types of Machine Learning:
Supervised Learning
Supervised Learning involves training a model on a labeled dataset. In this type of learning, the algorithm is provided with input data and corresponding desired output or labels. The goal is for the model to learn the relationship between the input and output so that it can make predictions on new, unseen data accurately. Supervised learning is used in tasks like image classification, spam email detection, and language translation.
Unsupervised Learning
Unsupervised Learning, on the other hand, doesn’t rely on labeled data. Instead, the algorithm explores the data’s inherent structure and identifies patterns or relationships. It’s particularly useful for tasks like clustering similar data points together or detecting anomalies within a dataset. Unsupervised Learning can help uncover hidden insights in data, making it a valuable tool in fields like market segmentation and recommendation systems.
Machine Learning algorithms, regardless of type, aim to minimize errors or discrepancies between predicted outcomes and actual outcomes during training. This iterative learning process allows models to improve their performance over time.
Deep Learning and Neural Networks
What are Neural Networks?
Artificial Intelligence Work: Deep Learning, a subset of Machine Learning, revolves around the use of neural networks. Neural networks are computational models inspired by the structure and function of the human brain. They consist of layers of interconnected nodes, known as neurons, that process and transform data. Each neuron takes input, processes it, and passes the result to the next layer. This layered structure allows neural networks to handle complex tasks, such as image recognition and natural language understanding.
Deep Learning vs. Traditional Machine Learning
Artificial Intelligence Work: Deep Learning excels in tasks that involve unstructured data, like images, audio, and text. It’s particularly powerful for tasks where feature engineering—explicitly selecting and transforming data attributes—is challenging or impractical. In contrast, traditional Machine Learning algorithms are more suitable for structured data and problems that can be solved through rule-based approaches.
Deep Learning has revolutionized AI, enabling breakthroughs in areas like speech recognition, autonomous vehicles, and healthcare diagnostics. It continues to push the boundaries of what machines can achieve by leveraging the capabilities of large neural networks and massive datasets.
In the next section, we will explore the critical role of data in Machine Learning and how it fuels the training of AI model
Data: The Fuel of AI
Data Collection and Preparation
Artificial Intelligence Work: To train AI models effectively, large amounts of data are required. Data collection involves gathering relevant information, while data preparation includes cleaning and formatting the data for analysis.
Feature Engineering
Feature engineering is the process of selecting and transforming the most important variables in the data to improve model performance.
The Role of Big Data
Artificial Intelligence Work: Big data technologies play a crucial role in AI by providing the infrastructure needed to store, process, and analyze massive datasets.
Training an AI Model
Training Data and Validation Data
Artificial Intelligence Work: AI models are trained on a subset of the data called the training data, while a separate dataset called validation data is used to assess the model’s performance.
Epochs and Iterations
Training involves multiple passes through the training data, known as epochs, with each pass refining the model’s parameters through iterations.
Algorithms in AI
Artificial Intelligence Work: AI relies on various algorithms to perform specific tasks. Some common algorithms include Linear Regression, Decision Trees, Random Forests, Support Vector Machines, and Clustering Algorithms.
Natural Language Processing (NLP)
Understanding NLP
Artificial Intelligence Work: Natural Language Processing is a branch of AI that focuses on the interaction between computers and human language. It enables machines to understand, interpret, and generate human language.
Sentiment Analysis
Sentiment analysis is a crucial application of NLP that determines the sentiment expressed in text, making it valuable for businesses to gauge customer opinions.
Language Translation
Artificial Intelligence Work: NLP also powers language translation services, allowing for accurate and efficient translation between languages.
Computer Vision
Image Recognition
Artificial Intelligence Work: Computer vision enables machines to interpret and understand the visual world, making tasks like image recognition and object detection possible.
Object Detection
Object detection algorithms identify and locate objects within images or videos, enabling applications such as autonomous vehicles and surveillance systems.
AI in Action
Artificial Intelligence Work: AI is already making a significant impact in various industries:
Self-Driving Cars
AI is at the core of self-driving car technology, allowing vehicles to perceive their surroundings and make driving decisions.
Healthcare Diagnostics
AI-powered diagnostic tools can analyze medical images, detect diseases, and assist healthcare professionals in making accurate diagnoses.
Recommendation Systems
Artificial Intelligence Work: Online platforms use AI to personalize recommendations, improving user experiences and driving engagement.
Ethical Considerations in AI
Artificial Intelligence Work: As AI becomes more prevalent, ethical considerations come to the forefront:
Bias and Fairness
AI algorithms can inherit biases present in training data, raising concerns about fairness and discrimination.
Privacy Concerns
Artificial Intelligence Work: The use of AI in data analysis and surveillance has sparked debates about individual privacy rights.
The Future of AI
Quantum Computing and AI
Artificial Intelligence Work: Quantum computing holds the potential to accelerate AI development by solving complex problems at unprecedented speeds.
AI’s Impact on the Job Market
AI’s automation capabilities may transform the job market, requiring a shift in workforce skills and roles.
Read More: Exploring the Metaverse in 2023: A Comprehensive Guide
Conclusion
In this comprehensive exploration of Artificial Intelligence (AI) and Machine Learning (ML), we’ve delved deep into the inner workings of these transformative technologies. We’ve uncovered the mechanisms behind AI’s ability to process data, learn from experience, and make informed decisions. From understanding the fundamentals of ML to the power of deep learning and neural networks, we’ve covered it all.
AI’s reliance on data has been emphasized throughout this article, highlighting the importance of quality data in training AI models. We’ve explored various types of Machine Learning, from supervised learning with labeled datasets to unsupervised learning’s capacity to discover hidden patterns within unstructured data.
FAQs
What is the difference between AI and Machine Learning?
AI is a broader field that encompasses the development of machines that can simulate human intelligence, while Machine Learning is a subset of AI that focuses on creating algorithms that allow machines to learn from data and make predictions or decisions based on that data. In essence, Machine Learning is a tool used within the field of AI to achieve intelligent behavior in machines.
How does AI affect everyday life?
AI has already made a significant impact on our daily lives. It powers virtual assistants like Siri and Alexa, recommendation systems on streaming platforms, and even traffic prediction in navigation apps. AI is also increasingly used in healthcare for diagnostics and personalized medicine. In essence, AI is making our lives more convenient and efficient.
Can AI replace human intelligence?
No, AI cannot replace human intelligence. While AI can perform specific tasks exceptionally well, it lacks the general intelligence, creativity, and emotional understanding that humans possess. AI operates based on predefined algorithms and data, whereas human intelligence involves complex cognitive processes and emotional awareness.
What are the limitations of AI?
AI has several limitations, including its dependence on data quality and quantity. It can also exhibit bias if the training data is biased. AI systems may not always be explainable, making it challenging to understand their decision-making processes. Additionally, AI cannot replicate human creativity and common-sense reasoning.
2 Comments