How to Implement Machine Learning in Financial Services
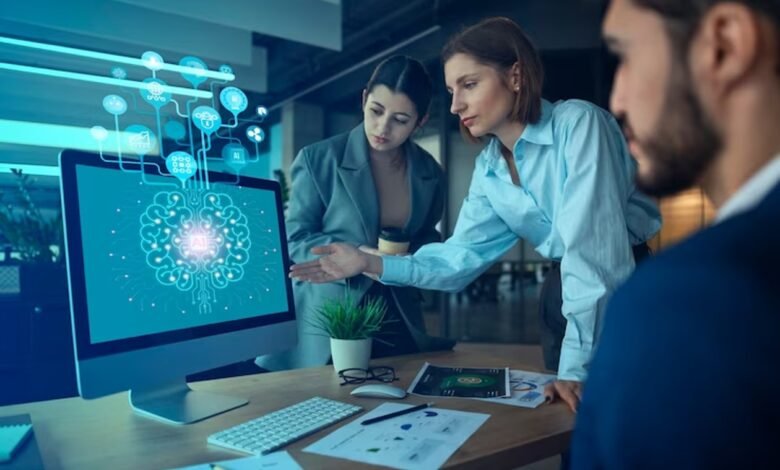
In the ever-evolving landscape of the financial services industry, staying competitive and meeting customer demands is crucial. One way to achieve this is through the implementation of machine learning. Machine learning has revolutionized various sectors, and the financial industry is no exception. This article explores the ins and outs of implementing machine learning in financial services.
Introduction to Machine Learning in Financial Services
What is machine learning?
Machine learning is a subset of artificial intelligence that involves the development of algorithms and statistical models that enable computer systems to improve their performance on a specific task through learning from data. In the context of financial services, it means utilizing these algorithms to make data-driven decisions, improve processes, and enhance customer experiences.
Why is it important in financial services?
The financial sector deals with massive amounts of data daily, and machine learning can analyze this data efficiently and accurately. It can detect trends, anomalies, and insights that would be challenging to identify with traditional methods.
Applications of Machine Learning in Finance
Machine Learning in Financial Services: Machine learning finds applications in various facets of financial services, and some of the prominent ones include:
Fraud detection
Machine learning models can identify fraudulent activities by analyzing transaction patterns and detecting anomalies in real-time. This proactive approach helps financial institutions save millions of dollars and protect their customers.
Risk assessment
By analyzing historical data and market trends, machine learning can assess credit risks, market risks, and operational risks, allowing financial institutions to make informed decisions and reduce losses.
Customer service improvement
Machine learning-powered chatbots and virtual assistants provide instant, round-the-clock customer support, enhancing customer satisfaction and reducing operational costs.
Read More: The Role of Machine Learning in Predictive Analytics 2023
Implementing Machine Learning in Financial Services
Machine Learning in Financial Services: Implementing machine learning in financial services involves several crucial steps:
Data collection and preprocessing
Gathering relevant data from various sources is the initial step. This data must be cleaned, transformed, and structured to ensure its suitability for analysis.
Model selection
Choosing the right machine learning model depends on the specific use case. Regression, classification, clustering, and deep learning models can be applied to solve various problems.
Model training and validation
Machine learning models must be trained on historical data and validated to ensure their accuracy and reliability. Fine-tuning the models is an iterative process that requires continuous improvement.
Deployment and monitoring
Once a model is ready, it is deployed into the operational environment. Continuous monitoring is essential to ensure that the model remains effective over time.
Benefits of Machine Learning in Financial Services
Machine Learning in Financial Services: The implementation of machine learning offers a multitude of benefits, including:
Enhanced efficiency
Automation of repetitive tasks and the ability to process vast amounts of data quickly lead to improved operational efficiency.
Improved accuracy
Machine learning models make predictions and decisions with a high degree of accuracy, reducing errors and associated costs.
Cost savings
Reduced fraud, improved risk assessment, and streamlined processes result in significant cost savings for financial institutions.
Challenges in Implementing Machine Learning
Machine Learning in Financial Services: Implementing machine learning in the financial services sector offers numerous benefits, but it is not without its share of challenges. These challenges need to be carefully addressed to ensure successful integration.
Data Privacy and Security
Data security is paramount in the financial industry, and when implementing machine learning, it’s crucial to handle sensitive customer data with the utmost care. Any breach or mishandling of data can result in severe consequences, both legally and in terms of trust. Robust security measures, such as encryption and access controls, are essential to protect sensitive financial information.
Regulatory Compliance
Financial institutions operate under a web of regulations and compliance requirements. When implementing machine learning, these regulations must be adhered to. Compliance with laws such as GDPR, HIPAA, and various financial regulations is essential. Machine learning models must be designed and trained with these requirements in mind, ensuring that data privacy is maintained, and all legal obligations are met.
Model Interpretability
Machine learning models, particularly deep learning models, are often considered as “black boxes.” They make predictions based on complex patterns and interactions within the data, making it challenging to understand how and why certain decisions are made. In the financial industry, model interpretability is critical, as regulators and stakeholders need to comprehend the reasoning behind significant decisions, especially those affecting financial transactions or risk assessments. Ensuring that machine learning models are interpretable and transparent is an ongoing challenge.
Data Quality and Quantity
Machine learning algorithms rely heavily on data. Ensuring the quality and sufficiency of data is a significant challenge. Incomplete, inaccurate, or biased data can lead to incorrect predictions and unreliable results. Data must be cleaned and preprocessed effectively to eliminate noise and ensure that the model learns from accurate information.
Talent Shortage
The financial industry is not only competing with other sectors for machine learning talent but also with technology giants and startups. Finding and retaining skilled data scientists and machine learning engineers can be a challenge. Financial institutions must offer competitive compensation packages and create an environment that fosters innovation to attract and retain top talent.
Scalability
As financial institutions grow, their data and computational needs also grow. Implementing machine learning solutions that can scale effectively to handle increasing data volumes and complexity is a challenge. This involves the selection of appropriate infrastructure, cloud services, and architectures that can adapt to evolving business needs.
Ethical Concerns
The use of machine learning in finance can raise ethical concerns, particularly when it comes to issues like algorithmic bias. Biased algorithms can lead to unfair treatment of certain customer groups, which can result in reputational damage and legal consequences. Ethical considerations are essential throughout the development and deployment of machine learning models in the financial sector.
Real-world Examples
Machine Learning in Financial Services: Several financial institutions have successfully implemented machine learning. For instance, J.P. Morgan uses machine learning to detect fraud, while Goldman Sachs utilizes it for algorithmic trading.
Best Practices
Machine Learning in Financial Services: To ensure a successful implementation of machine learning in financial services, consider the following best practices:
- Collaborate with data scientists and domain experts.
- Invest in data quality and governance.
- Continuously monitor and update machine learning models.
- Stay abreast of industry trends and emerging technologies.
The Future of Machine Learning in Finance
Machine Learning in Financial Services: The future of machine learning in finance is promising. As technology advances, machine learning will play an even more significant role in enhancing customer experiences, optimizing processes, and managing risks.
Read More: What is Data Analytics?
Conclusion
In conclusion, the implementation of machine learning in the realm of financial services represents a significant leap forward in the industry’s technological evolution. It offers a plethora of benefits, including improved efficiency, accuracy, and cost savings, which are paramount for institutions striving to stay competitive and meet customer demands.
Machine learning’s ability to analyze massive datasets in real-time, detect fraudulent activities, assess risks, and enhance customer experiences has transformed the financial landscape. It empowers financial institutions to make data-driven decisions, optimize operational processes, and deliver superior services to their clients.
However, the journey to harness the full potential of machine learning in financial services is not without its challenges. The challenges include ensuring data privacy and security, navigating complex regulatory requirements, and making machine learning models interpretable. Moreover, the quality and quantity of data, talent shortage, scalability, and ethical concerns all demand careful consideration.
FAQs
FAQ 1: Is machine learning widely used in the financial sector?
Yes, machine learning is widely adopted in the financial sector, with applications in fraud detection, risk assessment, and customer service improvement.
FAQ 2: What are the key challenges in implementing machine learning in finance?
The key challenges include data privacy and security, regulatory compliance, and ensuring model interpretability.
FAQ 3: Can small financial institutions benefit from machine learning?
Absolutely. Small financial institutions can benefit from machine learning by improving operational efficiency, reducing costs, and enhancing customer service.
FAQ 4: How does machine learning improve customer service in finance?
Machine learning-powered chatbots and virtual assistants provide instant and round-the-clock customer support, enhancing customer satisfaction.
FAQ 5: What are some regulatory considerations when implementing machine learning in financial services?
Financial institutions must ensure that their machine learning models comply with regulations such as data privacy laws and anti-money laundering rules.